Executive Summary
A leading global law firm faced the challenge of extracting specific valuable insights from a vast repository of legal documents. Traditional and out-of-the-box (OOTB) RAG solutions from hyperscalers proved inadequate for the level of complexity in the queries.
After unsuccessful attempts with traditional solutions, they partnered with Elastiq for a tailored RAG solution. This solution, combining various chunking strategies, a domain-specific knowledge layer, and an analytical engine that add capabilities beyond RAG, enabled them to solve for the complexities of the legal domain.
Client Profile
Our client is an American multinational white-shoe law firm with over 1,000 lawyers. The firm represents many of the world’s largest and most important public and private corporations, including a few Fortune 500 companies, asset managers, and financial institutions, as well as clients in need of pro bono assistance.
Business Problem
The law firm struggled to address complex legal queries, particularly those requiring document-specific analysis or analyzing all documents. Common challenges included:
- Complex document structures: Legal documents are complex and can be up to 100s of pages long in certain cases. When given questions like “What’s the discount provided to client X on their SOW regarding the matter Y?” chunking of the documents and maintaining relationships between chunks becomes critical.
In the above example, the name of the client, the discount information, and the matter information might all be in very different chunks. Hence, traditional semantic-search-based and traditional fixed-width-chunking-based RAG systems are not enough. Instead, enterprises need to build a domain-specific data structure for such a use case. - Analysis of information: RAG systems typically work on retrieval of information from source documents and can not “analyze” the information. For example, imagine a query like “What clients have their renewal coming up within the next 3 months?”
A traditional RAG system can not solve this question because none of the documents have the information about “renewal within the next 3 months”. Instead, the system should be able to understand the document expiry date for each contract and calculate whether the renewal will fall within the next 3 months. - Querying large datasets: While traditional RAG excels at retrieving relevant data from specific documents, it struggles to accurately generate responses where a large number of documents need to be analyzed. In other words, if the number of relevant embeddings for RAG exceeds the system’s top_k value, it can lead to incomplete, biased, or inaccurate responses.
For example: How many legal cases involving contract disputes were filed in California between 2018 and 2020? This might need 1000s of documents that need to be retrieved, analyzed, and counted, before responding.
The client evaluated various OOTB RAG solutions and failed! They needed a mature solution that could solve the above-mentioned challenges.
Elastiq Solution
Elastiq Discover – Elastiq’s advanced RAG platform offered a solution to these challenges:
- Smart Search: The platform’s ability to identify and focus on relevant document types (such as engagement letters, MSA, SOWs, etc.), Parties, Dates, and Clauses (amounts, discounts, etc.) enabled a smarter document chunk filtering on top of AI-based semantic search for content.
- Integrated Knowledge Layer: A backend data structure that builds relationships between different documents, document types, chunks, and various metadata related to various documents, clauses, and chunks. This enables the smart search mentioned above.
- Analytical Engine: An AI-based query engine that adds analytical capabilities to the knowledge layer. This allows the system to answer the queries that require analysis (aggregation, comparison, etc) of specific document metadata or clauses and not just retrieval.
Conclusion
A traditional OOTB RAG solution is great to get started with for a proof of concept with a very limited scope but is not enough to implement an enterprise-wide domain-specific AI solution.
With the level of complexity in documents and use cases in the real world for a domain (such as legal), it is critical to design an architecture from first principles that handles all complex scenarios. Elastiq Discover does that by building a knowledge layer and an analytical engine on top of RAG.
The solution helped the customer achieve operational efficiency by reducing document information retrieval time by up to 60%, demonstrating the power of a purpose-built AI approach.
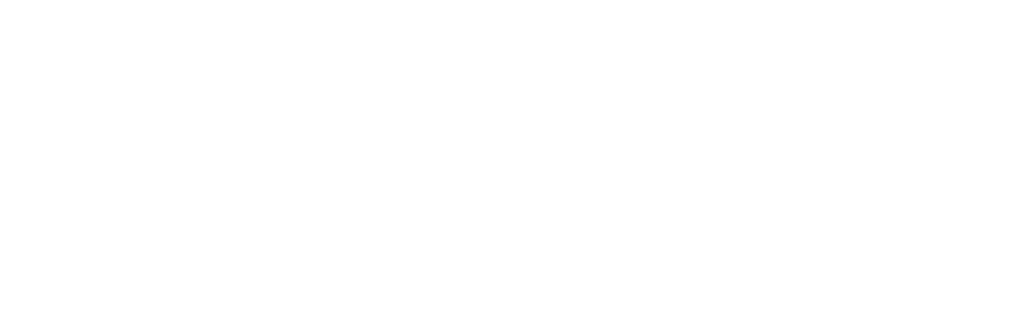
AI solutions for businesses